Water is one of the most precious resources on our planet, yet we often take it for granted. In...
Data-based strategies for adapting to climate change
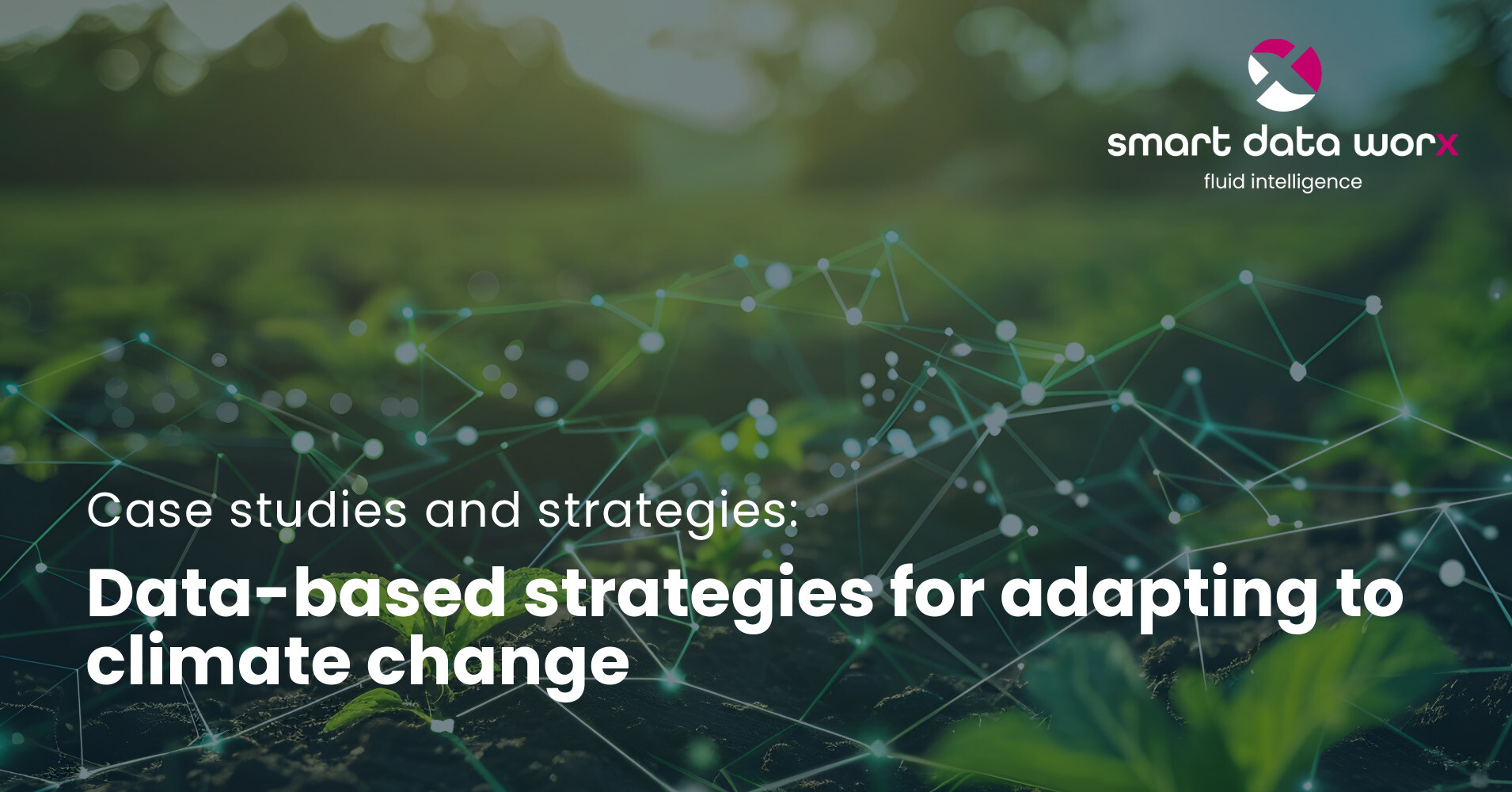
Climate change is one of the greatest challenges of the 21st century. The impacts are global and affect many aspects of human life and the natural environment. In order to effectively address these challenges, data-based climate change adaptation strategies are crucial. This article details how data analysis and AI-based modelling can be used to develop and implement adaptation strategies.
The use of data analysis to develop effective climate adaptation strategies
Importance of data collection and analysis
Data analysis plays a central role in the development of climate adaptation strategies. By collecting and analysing large amounts of data, patterns and trends can be identified that enable better predictions of future climate conditions and the planning of appropriate measures. This includes the use of meteorological data, environmental monitoring data, socio-economic data and other relevant information.
Data collection methods
Data collection is the first important step. This involves collating data from various sources:
- Satellite data: These provide large-scale information about the earth's surface, the sea surface and the atmosphere.
- Weather stations: They record local weather data such as temperature, precipitation and wind speed.
- Soil sensors: These measure soil temperature and moisture, which is particularly important for agriculture.
- Socio-economic data: This includes population statistics, economic indicators and health data.
Data management and processing
Once the data has been collected, it must be managed and processed. This includes cleansing the data, integrating different data sets and ensuring their quality and accuracy. Modern data management systems and cloud technologies play an important role here.
Data archiving is also an essential part of data management. It ensures that historical data is stored securely and is easily accessible when required. This is particularly important for compliance with legal regulations and for carrying out long-term analyses.
Analysis and modelling
The next step is to analyse the collected data. Various methods of data analysis are used here:
- Statistical analyses: These help to identify trends and patterns in the data.
- Machine learning (ML): ML algorithms can analyse large amounts of data and recognise complex correlations.
- Artificial intelligence (AI): AI models can be used to make predictions and simulate scenarios.
For example, analysing historical weather data can help to identify patterns and trends in climate change. This information can then be used to develop models that predict future climate conditions. Such models are essential for planning adaptation measures, as they make it possible to determine potential risks and opportunities.
Development of specific adaptation strategies
Based on the results of the data analysis, specific adaptation strategies can be developed. These strategies can be implemented in various areas, including
- Agriculture: adapting cultivation calendars, selecting climate-resistant plant varieties and optimising irrigation.
- Water resource management: planning water distribution, building water reservoirs and improving water utilisation efficiency.
- Urban planning: developing climate-resilient infrastructure, improving urban green spaces and adapting building regulations.
- Disaster prevention: development of early warning systems, emergency plans and risk management strategies.
Case studies
Agriculture in California
California is one of the most important agricultural regions in the world, but is also particularly susceptible to droughts. By analysing weather and soil data, scientists have developed models that can predict the water requirements and optimal irrigation time for different crops. These models help farmers to adapt their irrigation practices and use water more efficiently.
Flood protection in the Netherlands
Due to its geographical location, the Netherlands is particularly susceptible to flooding. By analysing historical flood data and developing AI-supported models, the authorities can make precise predictions about future flood risks. Based on these predictions, extensive flood defence measures have been implemented, including the construction of dykes, flood barriers and flood plains.
How AI-supported models can help mitigate the effects of climate change
More precise forecasts and scenario analyses
AI-supported models are able to analyse large amounts of data quickly and precisely. This enables more accurate predictions of climate patterns and extreme events. For example, AI models can be used to predict the probability and timing of heatwaves, storms or floods. These predictions are crucial for timely preparation and response to such events.
Development of innovative solutions
AI can also help to develop innovative solutions to mitigate the effects of climate change. Examples of this are:
- Energy efficiency in buildings: AI-supported systems can analyse energy consumption in buildings and make suggestions for optimisation. Significant energy savings can be achieved by adapting heating, ventilation and air conditioning systems.
- Intelligent electricity grids (smart grids): These use AI to optimise energy supply and demand in real time. This helps to ensure the stability of the electricity grid and increase the proportion of renewable energy.
- Sustainable mobility: AI can contribute to the development of traffic management systems that optimise traffic flow and reduce emissions. This also includes planning the charging infrastructure for electric vehicles.
Increasing the efficiency of adaptation measures
The use of AI can significantly increase the efficiency of adaptation measures. Examples of this are:
- Monitoring and controlling infrastructure: AI-supported systems can be used to monitor and control infrastructure in order to increase its resilience to the effects of climate change. This includes monitoring bridges, roads and dams in order to recognise potential damage at an early stage and initiate appropriate measures.
- Optimisation of irrigation systems in agriculture: By analysing weather and soil data, AI models can determine the optimal irrigation time and quantity. This leads to more efficient utilisation of water resources and increases resistance to periods of drought.
Case studies
AI-supported weather forecasts in India
India is particularly susceptible to extreme weather events due to its geographical location and population density. By using AI-powered weather forecasting models, Indian authorities can issue more accurate and timely warnings of storms, heatwaves and floods. This has helped to significantly improve preparedness and response to such events and save lives.
Optimisation of urban infrastructure in Singapore
Singapore is using AI to optimise its urban infrastructure and tackle the challenges of climate change. AI-supported systems monitor water consumption, the energy efficiency of buildings and traffic flow. These measures have enabled Singapore to increase its resilience to the effects of climate change while achieving its environmental goals.
Conclusion
Adaptation to climate change requires innovative and data-based strategies. The use of data analysis and AI-supported models offers enormous potential. By collecting, analysing and using relevant data, well-founded decisions can be made and efficient measures developed. AI-supported models enable more accurate forecasts, the development of innovative solutions and an increase in the efficiency of adaptation measures. Overall, these approaches help to mitigate the effects of climate change and strengthen the resilience of our society and environment.
The combination of data analytics and AI creates a solid foundation for the development of sustainable and adaptive solutions that can help us effectively address the challenges of climate change. By utilising and advancing these technologies, we can build a more resilient and sustainable future.